The advancements made in multi-omics technologies have enabled molecular biologists to investigate cellular and molecular events in unparalleled detail, offering a comprehensive and holistic tool for exploring biological systems. Up until now, this level of resolution has not been possible to apply to tissue samples, limiting the contextual profiling of cells within their microenvironment.
Enter spatial biology, which adds a new powerful layer to existing analysis tools by teasing out the activities of individual cells within their spatial architecture. For diseases like cancer, this level of resolution is critical for understanding the tumour microenvironment (TME) and the interaction of different cells within this context. The specific locations of cells or molecules within a highly organized biological system, coupled with high dimensional data, is crucial for pathophysiology, diagnosis, prognosis, and the ultimate management of patients with altered responses to therapy.
Spatial Biology for Immuno-oncology: The Immune Landscape
The patterns by which tumours evolve during metastatic progression and the resulting clinical outcomes for patients with cancer are influenced by the immune environment at the metastatic site. Understanding the immune makeup of a tumour, including the concentration, composition, functional state, and arrangement of immune cells infiltrating it, can offer valuable insights for predicting how a patient will respond to treatment and what their prognosis might be. Many of the pioneers of spatial biology come from the cancer field and have demonstrated key areas where this technology can provide critical information about why some cancer patients are cured by immuno-oncology treatments, whilst others showed little benefit. In particular, the advancement of multiplex immunofluorescence (mIF) technologies has made it possible to analyze the TME in greater detail. This technology can define the immune contexture by quantitatively evaluating the types of immune cells present, their functional characteristics, and how they are positioned within the TME, thus providing crucial contextual and spatial information. Unveiling the tumour microenvironment in exquisite detail has been a game changer to understanding the biology of cancer, and the response of the immune system during clinical interventions.
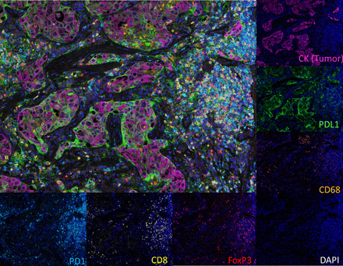
You’d be hard pressed to speak about spatial biology for cancer without mentioning the seminal work done by immunologist Jerome Galon from the INSERM Laboratory of Integrative Cancer Immunology. His laboratory has identified and demonstrated the importance of the immune contexture when considering cancer treatment. Through several high impact studies, Dr. Galon has demonstrated the value of in situ analysis of tumour-infiltrating immune cells as a prognostic tool for a variety of cancers. The first paper, published in Science in 2006 has been cited over 6000 times and since then, numerous analyses of large patient cohorts have been conducted and successfully identified specific patterns of immune activation associated with patient survival. This led to the establishment of the “Immunoscore”, a standardized, immune stratification system encompassing the type, functional orientation, density, and location of adaptive immune cells within distinct tumour regions, providing a new method for routine clinical assessment of prognosis of cancer patients.
The Immunoscore
The Immunoscore is based on the quantification of two lymphocyte populations (CD3/CD45RO, CD3/CD8 or CD8/CD45RO), both in the core of the tumour (CT) and in the invasive margin (IM). The Immunoscore provides a scoring system ranging from low immune cell densities found at both the CT and the IM, Immunoscore 0 (I0), to high densities classed as Immunoscore 4 (I4), with increasing scores correlating with longer patient survival.
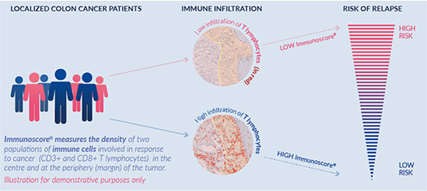
In the clinic
Prediction of clinical outcomes in cancer has historically been achieved by histopathological evaluation of tissue samples obtained during surgical resection of the primary tumour. The conventional staging of tumours (referred to as AJCC/UICC-TNM classification) compiles information about the size of the tumour (T), the presence of cancer cells in nearby lymph nodes (N), and indications of metastasis (M). The accumulating data from spatial biology studies from large cohorts of human cancers has indicated that spatial classification as a prognostic value may add to the AJCC/UICC TNM classification.
In an effort to promote the utilization of the Immunoscore in routine clinical practice, the Society for Immunotherapy of Cancer initiated the Immunoscore validation project. In 2018, findings were published in the Lancet with an article entitled “International Validation of the Consensus Immunoscore for the Classification of Colon Cancer: A Prognostic and Accuracy Study”. This large, multi-site study demonstrated the utility of the consensus Immunoscore assay in 2681 patient samples with TNM stage I-III colon cancer.
The Immunoscore assay showed a high level of reproducibility between observers and centres (r=0·97 for colon tumour; r=0·97 for invasive margin; p<0·0001). In the training set, patients with a high Immunoscore had the lowest risk of recurrence at 5 years (14 [8%] patients with a high Immunoscore vs 65 (19%) patients with an intermediate Immunoscore vs 51 (32%) patients with a low Immunoscore). The findings were confirmed in two validation sets (n=1981). The Immunoscore had the highest relative contribution to the risk of all clinical parameters, including AJCC/UICC TNM classification system. Overall, the Immunoscore provided a reliable estimate of the risk of recurrence in patients with colon cancer and supports the implementation of the consensus Immunoscore as a new component of a TNM-Immune classification of colon cancer. The Immunoscore has not yet been validated for other malignancies.
Beyond colon cancer
There are a few limitations to applying the Immunoscore for other malignancies, especially when biopsies from metastases, and not resected tumours, are obtained where the IM is rarely available, which is often the case in clinical trials. Nevertheless, the advances made in characterizing the immune landscape at the TME have demonstrated the utility of spatial biology for colon cancer prognosis and research in this field is advancing at a rapid pace. There are major studies underway to fully understand the TME in various malignancies to provide additional spatial scores for patient stratification. Of course, this does not exclude the evaluation of other markers. Whilst T-lymphocytes are currently the focus of oncology research, other cell types could provide a greater understanding of the TME. For example, tumour-infiltrating B-cells and the so-called tertiary lymphoid structures resembling lymph nodes, are of great interest since their mechanism of interaction with the tumour and TME have a crucial, synergistic role in tumour control. Predicting responses to immune checkpoint inhibitors (ICI) is another avenue where spatial biology can be useful, where spatial phenotyping methods measuring “hot” and “cold” tumours can explain the clinical responses to ICIs. In this model, cancers are differentiated by expression of T-cell markers and interferon (IFN) signatures, regardless of their mutational status. Measuring the density of T-cells in the TME, or their activation status, has proven to outperform stand-alone PD-L1 IHC and other biomarker tests in anti-PD-L1/PD-1 immunotherapy response prediction. The development of novel biomarkers for spatial biology is clearly needed and this is an active area of research. In addition to biomarkers and methods directly involving the TME, the parallel assessment of circulating components should also be considered.
Choosing the right spatial biology tool
With the explosion of spatial biology platforms, it can be difficult to settle on an appropriate method for a spatial biology study. In general, spatial biology can be divided into two categories, spatial transcriptomics and spatial proteomics (with a special mention for spatial metabolomics which uses a completely different platform and is beyond the scope of this article), with some platforms able to measure both modalities in one system.
There are several factors that need to be considered when choosing an application, dependent on the project type, the research objectives and the specific sample type being analyzed:
- Target of interest
Both the transcriptome and proteome play a key role in disease and treatment response. Although transcripts provide gene expression data at a given point in time, as proteins are the molecular effectors of the cell, these can provide a more accurate phenotypic picture of molecular events. Ideally, a multi-omic platform that can integrate both transcriptomic and proteomic data will provide the highest level of understanding in tissue biology, bearing in mind that costs will substantially increase, and workflows will be complex.
- Resolution and sensitivity
Different spatial biology platforms have slightly different techniques to resolve location and thus, resolution can vary quite substantially across platforms. Generally, microscopy-based systems that can directly image the tissue sample are able to produce the highest level of resolution. The highest resolution systems can resolve gene or protein expression at the sub-cellular level but at the expense of larger fields of view. Where high resolution is not required, tools that utilize barcodes to map elements back to their original locations via sequencing readouts to generate an image may be used. In this case, 55–100-micron resolution is achieved, and results can be seen as a view of a “cellular neighbourhood”. Some researchers may be interested in rare biomarkers, or a single transcript copy in a cell, and so should look for applications where the sensitivity is high. Generally, direct detection strategies like single-molecule fluorescence in situ hybridization will perform better than indirect detection strategies.
- Multiplexing
One of the biggest differentiators among spatial biology platforms is their capacity, both for the number of analytes that can be queried and for the number of samples that can be analyzed simultaneously. For true discovery science, where there is no prior knowledge about biomarkers or biological activity and all proteins or all genes need to be considered, a platform with the highest level of multiplexing is necessary. For translational science, where a core set of genes or proteins are already defined, the processing and analysis can be streamlined allowing faster generation of results for a fraction of the price. Usually, the detection of dozens of proteins and up to 100 genes may be detected. In a clinical setting, a subset of genes or proteins is sufficient and more practical to implement, with the ability to run several samples at once.
Article written by Caroline Beltran
References
- Pagès. F., et al. 2018. International validation of the consensus Immunoscore for the classification of colon cancer: a prognostic and accuracy study. The Lancet. DOI: 10.1016/s0140-6736(18)30789-x
- Galon, J., et al. 2017. World-Wide Immunoscore Task Force: meeting report from the “Melanoma Bridge”. Journal of Translational Medicine. DOI 10.1186/s12967-017-1310-9
- Galon, J., et al. 2012. Cancer classification using the Immunoscore: a worldwide task force. Journal of Translational Medicine. DOI: 10.1186/1479-5876-10-205
- Pagès, F., et al. 2009. In situ cytotoxic and memory T cells predict outcome in patients with early-stage colorectal cancer. Journal of Clinical Oncology. DOI: 10.1200/JCO.2008.19.6147
- Marliot, F., et al., 2020. Analytical validation of the Immunoscore and its associated prognostic value in patients with colon cancer. Journal for Immunotherapy of Cancer. DOI: :10.1136/jitc-2019-000272
- Laumont, C. M., 2022. Tumour-infiltrating B cells: immunological mechanisms, clinical impact and therapeutic opportunities. Nature Reviews Cancer. DOI: 10.1038/s41568-022-00466-1
- Deutsh, J. S., et al., 2023. Combinatorial biomarker for predicting outcomes to anti-PD-1 therapy in patients with metastatic clear cell renal cell carcinoma. Cell Reports Medicine. DOI: 10.1016/j.xcrm.2023.100947
- Galon, J., et al. 2023. The Continuum of Cancer Immunosurveillance: Prognostic, Predictive, and Mechanistic Signatures. Immunity Reviews. DOI: 10.1016/j.xcrm.2023.100947
- Binnewies, M., et al., 2018. Understanding the tumor immune microenvironment (TIME) for effective therapy. Nature Medicine. DOI: 10.1038/s41591-018-0014-x
- https://www.sitcancer.org/research/immunoscore